Create a free profile to get unlimited access to exclusive videos, sweepstakes, and more!
How close are we to Free Guy's digital awareness? The Science Behind the Fiction
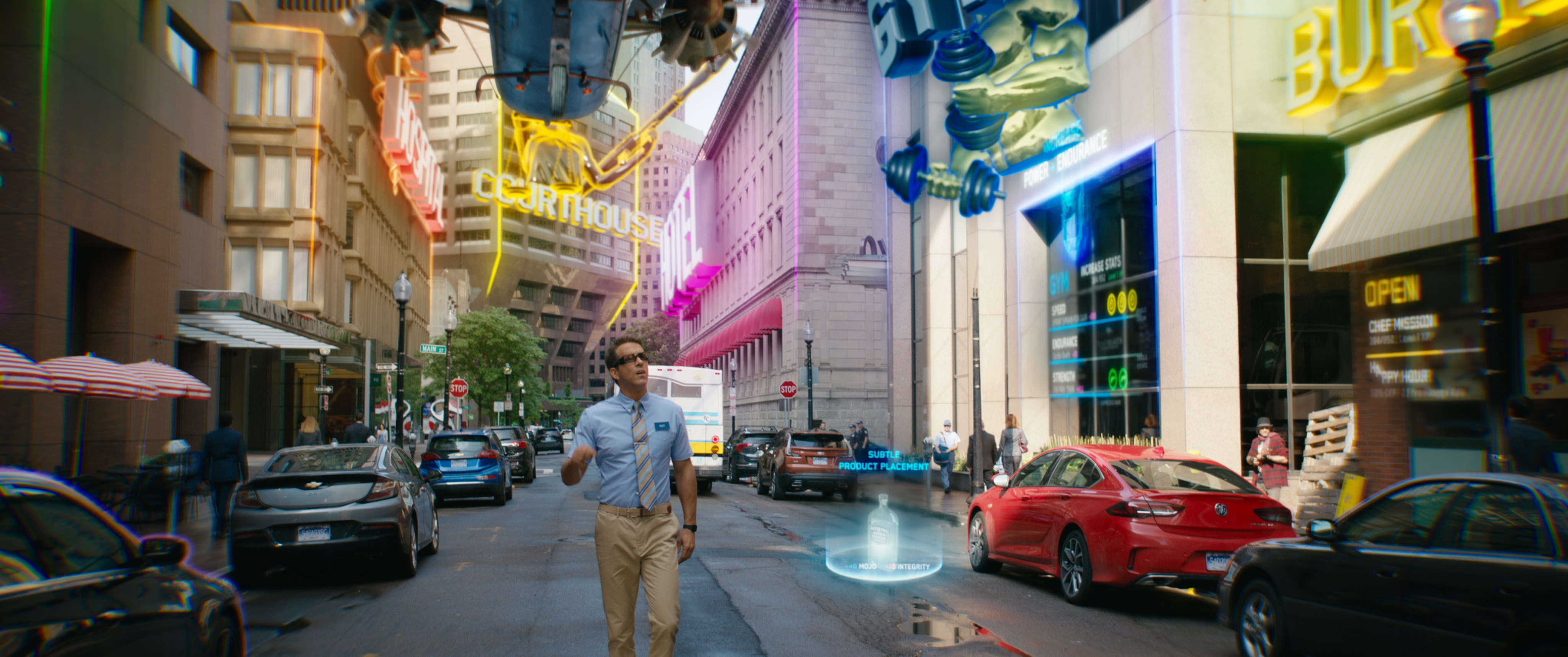
Free Guy bills itself as a comedy, but it exists in a world in which Ryan Reynolds doesn’t exist. Which, of course, makes it a tragedy. The movie solves this problem by building its own Ryan Reynolds out of code, inside a video game. A reasonable response to such a terrible lack. But, Guy (Reynolds) is more than just a simple NPC. He's self-aware and, in a way, alive.
In truth, the emergence of Guy as a fully-fledged awareness inside the game wasn’t wholly directed. Instead, he blossomed from a set of prior conditions, much like the IOs in Tron Legacy, having learned from his experiences inside the game environment.
If anyone has yet built living entities out of zeroes and ones, they’re keeping that information to themselves (a terrifying prospect) but artificial intelligences have long been a staple of video games. And they’re getting smarter.
WOULD YOU LIKE TO PLAY A GAME?
For decades, video games have been a ready benchmark for testing the latest artificial intelligences. First, we need a quick primer in defining terms. While the term "artificial intelligence" conjures images of replicants and Skynet, it can refer to any number of systems designed to help a computer or machine complete a task.
The simplest of these systems is reactive; a machine takes in a set of conditions and, based on its programming, determines an action. These sorts of AI are common in video games. An enemy may attack once you’ve entered into a pre-determined perimeter then, depending on conditions set by the game designers, its behavior plays out. Maybe it continues to attack until you or it are defeated. Maybe it attacks until its health bar reaches a critical low and then retreats.
To the player, the game character appears to be making decisions, even while it’s essentially navigating a flowchart. And this sort of AI will behave in the more-or-less the same way each time you encounter it. It isn’t thinking about what happened in the past or what might happen in the future, it’s simply taking existing conditions, bumping them up against potential actions, and selecting from those available.
It could be argued that these types of AI have existed since the dawn of video games. Even the computer opponent in Pong took a measure of the playing field and altered position in order to better defend the ball. Were that not the case, the opposing cursor would simply move randomly along the field, and the game would be no fun.
More advanced AI have limited memory, they store at least some of their past interactions and use that knowledge to modify future behavior. This is closer to what we think of when we think of AI, a machine that not only thinks, but learns and then thinks differently.
Much was made of the Nemesis system in Warner Bros.’ Middle-earth: Shadow of Mordor, when the game dropped. Instead of seemingly brainless adversaries which could be defeated through sheer force of will, Shadow of Mordor offered something closer to life. Enemies remember you, hold grudges, and alter their tactics based on yours. They have limited memory, and they learn from you. It made for a different sort of game-play experience by making the other characters a little more real.
In 2019 Google’s AlphaStar AI, built by their DeepMind division, set about rising the ranks of StarCraft II. The folks at DeepMind chose StarCraft because of its complexity when compared to games like chess.
Chess, with its comparatively limited tokens and move-types, still boasts a truly staggering number of possibilities. StarCraft ratches up the complexity, making it a reasonable next step for game AI. The team started by feeding AlphaStar roughly a million games played by human players. Next, they created an artificial league, pitting versions of AlphaStar against one another. The system learned.
Eventually, AlphaStar was let loose on some of the best StarCraft players in the world and quickly rose in the ranks. While it didn’t beat everyone, it did place in the top half-a-percent, and that’s even with its speed capped to match what humans are capable of. All of this was possible due to AlphaStar’s ability to take in information and learn from it, refining its process as it goes.
Most modern AI are built on this model. They take in a data set, either provided in advance or learned through interaction, and use it to build a model of the world. Or, at least a model of the thin slice of the world with which they are concerned. Image detection programs are trained on previously viewed images. They look for patterns and, over time, get better at recognizing novel images for what they are. Chatbots do something similar, cataloging the various conversations they have with people or with other bots, to improve their responses. Each of these programs can become skilled at limited tasks, matching or even exceeding human ability.
Those limits, the boundaries within which AI operate, are precisely what make them good at their jobs. It’s also what prevents them from awareness. AlphaStar might be good at StarCraft, but it doesn’t enjoy the thrill of victory. For that, we’d need…
THEORY OF MIND AND FULL AWARENESS
We can’t get a robot uprising, a mechanical Haley Joel Osment, or a digital Ryan Reynolds without stepping up our game. Building truly intelligent machines requires that they have a theory of mind, meaning they understand there are other thinking entities with feelings and intentions.
This understanding is critical to cooperation and requires a machine to not only understand a specific task, but to more fully understand the world around them. Today, even the best AI are operating solely from the information they’ve been given or have gleaned from interaction.
The goal is to have machines capable of taking in the complex and seemingly random information in the real world and making decisions similar to the ones we would make. Instead of delivering commands, they would pick up on social queues, unspoken human behavior, and unpredictable chance occurrences. We, likewise, should be able to have some understanding of their experience — if it can be called experience — if we hope to collaborate meaningfully.
Alan Winfield, a professor of robot ethics at the University of West England, suggests one way of accomplishing an artificial theory of mind. By allowing machines to run internal simulations of themselves and other actors (machines and humans), they might be able to play out potential futures and their consequences. In this way, they might gain an understanding that other entities exist, have motives and intentions, and how to parse them.
One major difficulty is the stark reality that we don’t really understand theory of mind even in ourselves. So much of what our brains do happens behind the scenes, and it’s the amalgamation of those processes which likely result in awareness.
This, too, is the major hurdle in designing machines with full awareness. But pushing toward a machine theory of mind might help us get closer. If human awareness is an emergent property of countless simpler processes all working together, that might also be the path for achieving awareness in machines or programs. Not with a flash, but slowly and by degrees.
True artificial intelligence of the kind seen in novels, movies, and television shows is probably a long way off but the seeds may already be planted, maybe even in a video game you've been playing.